Generalization Bounds for Causal Regression
Work done in collaboration with Claudio José Struchiner and Guilherme Tegoni Goedert.
In causal regression, we seek to make predictions that estimate the effects of interventions. In particular, we try to reason about what would happen, rather than what hapenned. This is very tricky, because we don’t have access to what would have happened for a certain individual under an intervention if that intervention did not happen.
Many algorithms have been proposed to predict causal quantities such as potential outcomes and treatment effects. However, there is no backing theory. And moreover, because we don’t have access to the true counterfactuals in observational data, it’s impossible to do any faithful benchmarking — so we really need theory here!
In this work, we establish some fundamental and general results for achieving strong guarantees on modern causal prediction methods. Our methods can be easily adapted to new algorithms, naturally adjusting to their intricacies.
Our main result is a bound relating the unobservable causal quantity to its observable analogue, bounding their gap as a function of the variance of the treatment propensities over the population (taking into account potentially unobserved confounders):
Moreover, we show that this variance term can be — somewhat surprisingly — upper bounded by a quantity that can be estimated in practice regardless of any unobserved confounders, as a function of the quality of a treatment assignment classification model (also known as a propensity scoring model):
With both of these inequalities in hand, we can connect them with generalization bounds for base algorithms. We give an example in the paper with generalization bounds based on the Rademacher complexity, but the same approach works for pretty much any existing generalization bound (e.g., those based on VC dimension, algorithmic stability, PAC-Bayes, etc.).
We also prove the analogous results for treatment effect estimation for a wide family of models (T-learners, S-learners and X-learners), which can be naturally extended to even more situations.
Our bounds are remarkably tight, vastly outperforming the closest matching bounds previously available in the literature, which is several orders of magnitude looser (!).
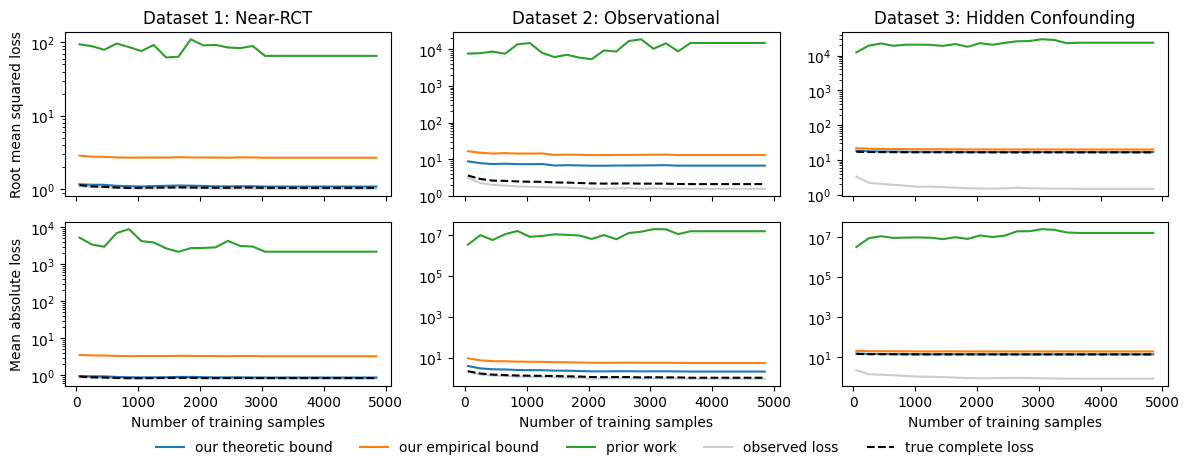
Comparison between our bounds and those of the closest matching prior work on three datasets of increasing complexity. Our “theoric” and “empirical” bounds correspond to the two bounds presented above, respectively. Our theoric bound is quite tight, being very close to the complete loss (which is unobservable in practice). Our empirical bound, while somewhat looser than the theoric bound, is still substantially tighter than the available prior work.
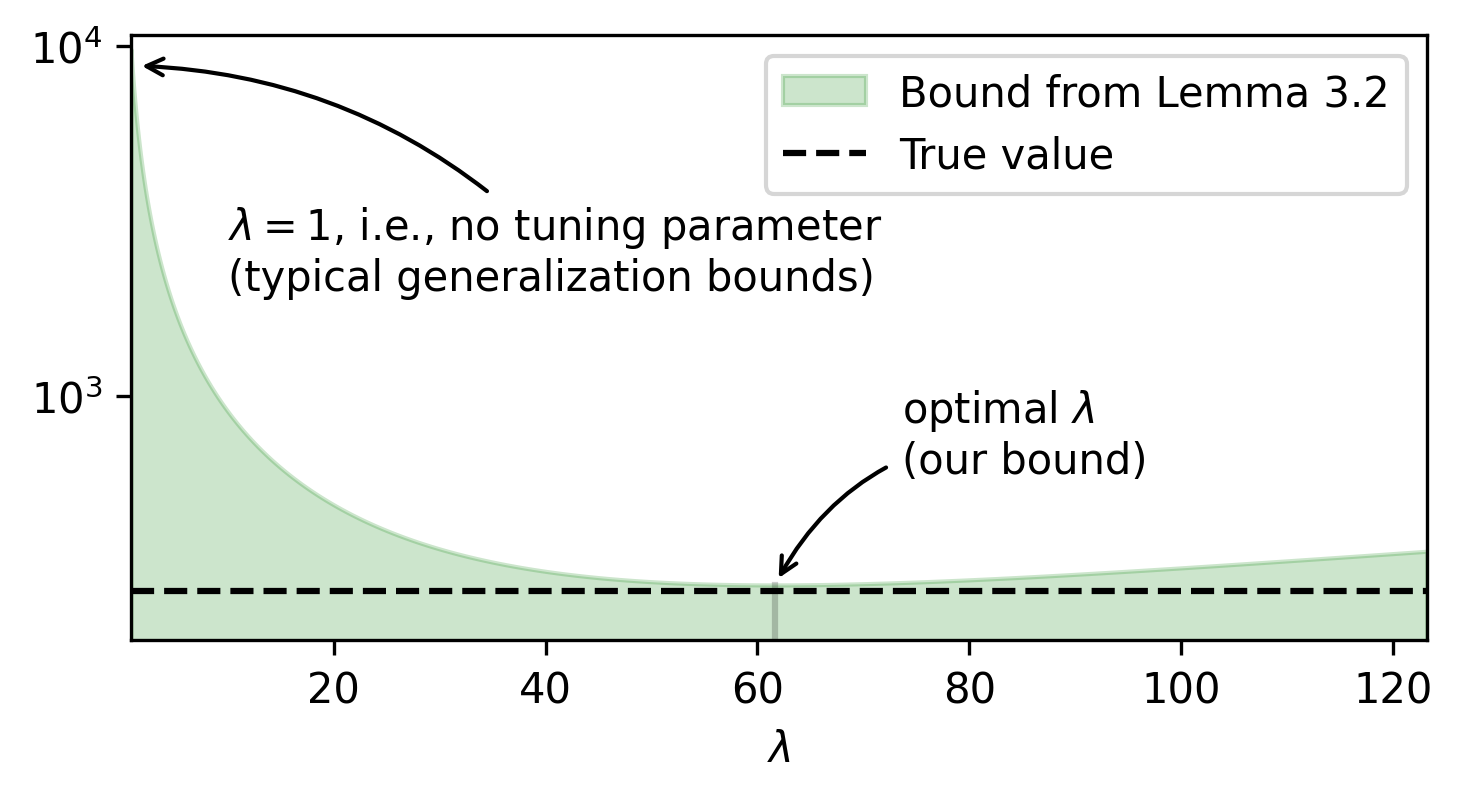
A key contributor to the tightness of our bounds is the parameter, which can be tuned for better bounds. Previously existing bounds roughly correspond to taking , and taking other values of can can lead to bounds that are orders of magnitude tighter.
Full details in the paper! You can find it here and on ArXiv.